Translate this page into:
DRRGenerator: A Three-dimensional Slicer Extension for the Rapid and Easy Development of Digitally Reconstructed Radiographs
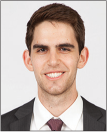
*Corresponding author: Lance Levine, University of Miami Miller School of Medicine, Miami, Florida, United States. l.levine4@med.miami.edu
-
Received: ,
Accepted: ,
How to cite this article: Levine L, Levine M. DRRGenerator: A Three-dimensional Slicer Extension for the Rapid and Easy Development of Digitally Reconstructed Radiographs. J Clin Imaging Sci 2020;10:69.
Abstract
As the interest in image-guided medical interventions has increased, so too has the necessity for open-source software tools to provide the required capabilities without exorbitant costs. A common issue encountered in these procedures is the need to compare computed tomography (CT) data with X-ray data, for example, to compare pre-operative CT imaging with intraoperative X-rays. A software approach to solve this dilemma is the production of digitally reconstructed radiographs (DRRs) which computationally simulate an X-ray-type image from CT data. The resultant image can be easily compared to an X-ray image and can provide valuable clinical information, such as small anatomical changes that have occurred between the pre-operative and operative imaging (i.e., vertebral positioning). To provide an easy way for clinicians to make their own DRRs, we propose DRR generator, a customizable extension for the open-source medical imaging application three-dimensional (3D) Slicer. DRR generator provides rapid computation of DRRs through a highly customizable user interface. This extension provides end-users a free, open-source, and reliable way of generating DRRs. This program is integrated within 3D Slicer and thus can utilize its powerful imaging tools to provide a comprehensive segmentation and registration application for clinicians and researchers. DRR generator is available for download through 3D Slicer’s in-app extension manager and requires no additional software.
Keywords
Digitally reconstructed radiographs
Slicer
X-ray
Computed tomography
INTRODUCTION
Despite image-guided operations composing a larger percentage of all medical procedures, the necessary analysis software is currently not widely affordable or accessible. Most radiology analysis is done using very expensive toolkits and in-house implementation of the necessary algorithms. These commercial software applications have limited extensibility and may impose limitations on their usage. One crucial component of these programs is the generation of digitally reconstructed radiographs (DRRs).
DRRs are simulated radiographic images produced through perspective projection of a three-dimensional (3D) image (volume) onto a two-dimensional (2D) image plane.[1] The typical example of a DRR would be to simulate an X-ray image from a computed tomography (CT) volume.[2] The simulated X-ray would have benefits over traditional X-rays in a variety of ways. DRRs can produce images from any angle on the fly, as well as alter parameters such as emitter distance that would not be practically feasible with traditional X-rays. An emerging field of using DRRs in a clinical setting is to allow physicians the ability to continuously monitor the position of the patient during the medical procedure using 3D imaging technology. For example, a pre-operative CT can be used to generate DRRs which can then be registered with X-rays acquired during an operative procedure. By registering the real and simulated X-rays, valuable information can be rapidly acquired during the operation such as location of structures accounting for small changes in patient positioning. This process is swift and accurate enough to be used for online clinical applications.[2]
Many DRR generation algorithms have been proposed – such as cylindrical harmonics,[3] reassembling previously generated projection blocks,[4] and GPU acceleration[5] – but none of these provide a program that end-users can download and use in their analyses. Programs that use DRRs utilize them as part of their registration workflow, but do not allow generation of DRRs, which provide independent clinical value. For example, plastimatch possesses a 3D Slicer extension that utilizes DRRs under the hood, but has no options for allowing the user to generate their own DRRs. There is not a widely available, free DRR generator that depends only on open-source software. Proposed in this paper is an extension for 3D Slicer named DRR generator that allows the rapid and accurate generation of DRRs. 3D Slicer is free and open source and provides extensive flexibility, frequent updates, support for many other common data formats, and revolutionary algorithm toolkits.[6]
MATERIAL AND METHODS
Many of the necessary components, such as visualization of anatomical images, are already included natively in 3D Slicer. An extension for 3D Slicer was written that allows for the rapid generation of DRRs using a highly customizable graphical user interface.
Protocol
Platform
This extension was developed for the popular scientific imaging platform 3D Slicer. 3D Slicer provides an abundance of useful tools to analyze and represent virtually every type of scientific data set, including interactive segmentation, registration, and volume rendering. It has features that can be used for every organ in the body and is compatible with all common imaging modalities. It also allows for a bidirectional interface for devices, making it a one-stop-shop for clinicians and researchers to develop and test new medical technologies. It provides much of the same functionality as commercial programs while remaining free and open source. 3D Slicer allows unrestricted use for all users under a BSD-style license,[7] even for commercial uses. It is designed to allow for custom modules to be easily integrated with full access to the underlying scientific toolkits, which can be distributed and then downloaded from within Slicer itself. The most recent version, Slicer4, has been downloaded hundreds of thousands of times all across the world.
Design
3D Slicer provides numerous ways to implement custom modules that provide different levels of integration and even utilize different programming languages. To allow for full accessibility of internal toolkits (such as ITK, a powerful toolkit for scientific image analysis), a loadable module was developed, meaning the code was written in C++ and was integrated closely with Slicer. This module type required building the 3D Slicer application from its source code and integrating the extension into the actual build. DRR generator is implemented as one extension, containing one module that provides all the functionality. Visualization toolkit (VTK) is an open-source software for 3D computer graphics that utilizes C++ and has several interface levels including Java and Python.[8]
The extension operates by virtually simulating the way an X-ray works. First, the data are imported and converted to a VTK image, where the image can be rotated, translated, and scaled as desired using the graphical user interface [Figure 1]. A virtual X-ray source is then established, which can also be customized with regard to position. A 2D image is generated, with each pixel corresponding to the equation of the corresponding ray that will be cast to it through the CT image. The source and DRR image move as one unit to simulate the rigidity of the X-ray gantry. A threshold (that the user may adjust) is established, above which the intensity values of the CT will be integrated into the DRR. A bilinear interpolation is then performed to integrate each plane of traversed voxels along each ray. This is a combination of two linear interpolations, first along the x-axis and then along the y-axis, so that the resultant value is a calculation of both the normalized and relative X-ray intensity of each ray passing through the CT. The bilinear interpolation is represented as
f(x, y) ≈ a0+a1x+a2y+a3xy
where the coefficients are obtained by solving
Where, x and y are adjacent pixels. The resultant DRR then has its intensity values normalized and is stored as one slice of a different 3D VTK volume. This volume is simply a 3D volume containing the 2D DRR slice, which may be passed to any desired VTK filter to change the visual appearance of the DRR. The result is then outputted to a Slicer volume node for further analysis and/or visualization.

- A flowchart depicting the digitally reconstructed radiograph generation algorithm.
Implementation
DRR generator is written in C++ and is integrated tightly into the core of 3D Slicer. This provides faster processing than would be possible with other ways to design modules, such as writing python modules that have less access to the full data processing resources of 3D Slicer. This also provides the ability to utilize the DRR generator as a standalone program that does not require the 3D Slicer run-time environment. This program can be run on Windows, Linux, and Mac OS X operating systems.
DRR generator uses the native DICOM importer for 3D Slicer to import medical images [Figure 2]. The user may then modify parameters they would like to specify for their DRR, such as the translation, rotation, and scale of the image, and the distance from the emission source to the image. The DRR can then be generated to overwrite an existing volume or to generate a new 2D volume containing the DRR image data.
Case 1: Vertebral positioning during surgery
Numerous procedures require interfacing with vertebrae, from spinal surgery to tumor ablation. Certain pathologies, such as hepatocellular carcinoma of the liver, are best accessed through the back to reach the tumor adequately and to limit damage to surrounding structures. Pre-operative imaging is used in this case to plan the proper trajectory of the ablation needle, but positioning on the operating table may alter the alignment of the individual vertebral segments from the pre-op imaging. In this scenario, intraoperative X-rays could be taken to analyze the new vertebral positions, but cannot be compared to the pre-operative CT directly. For this comparison to occur, a DRR must be generated from the pre-operative CT data to compare the X-ray with a simulated X-ray. The changes in position can then be grossly accounted for so the patient can be readjusted to a more suitable position.
Case 2: Catheter reconstruction in brachytherapy
Brachytherapy is a cancer treatment that involves inserting radioactive sources into a patient to destroy cells and tumors. It is common for physicians to use catheters filled with radioactive material for insertion near the tumor. Catheter reconstruction for position placement in high-dose rate brachytherapy in the past has been accomplished by methods such as flat panel detectors[9] and electromagnetic 3D tracking systems.[10] More recently, the construction of DRRs from CT data has become a faster and more accurate way of determining where to place the catheter. CT data are very useful because it provides accurate size and position of the target area. By generating a DRR of the prostate region, it is now possible to determine the best gantry angles for catheter insertion.[11] Using conventional film acquisition, the patient is continually exposed to radiation, while the DRR calculation requires no additional patient irradiation. The production of DRRs also allows for filtering between geometric and anatomical views. If the area adjacent to the prostate designated for catheter insertion is surrounded by bone, switching to the anatomical filter would allow bone to be replaced by soft tissue for easier viewing.
Case 3: Enhanced visualization of structures
Projected anatomy poses a limitation to radiographs. Patient anatomy can inhibit the detection of certain lesions (such as lung nodules) or can lead to the skewing in appearance of structures by those around it. DRRs have far less noise and can aid in these visualizations, as well as providing different angles to attempt visualization. By modifying the parameters and contrast, one can generate a DRR that demonstrates outer physical appearance, which can aid in such scenarios as identifying deceased bodies using past CT data.

- An example of a digitally reconstructed radiograph (DRR) generation. The top left quadrant shows the DRR, the bottom quadrants show different views of a computed tomography image, and the top right quadrant shows where the data can be visualized in three-dimensional space. Window/level is set to differentiate bone by default, but can be modified within Slicer to change the contrast of the image. Noise can be added to further simulate a traditional radiograph.
Validation
The accuracy of this program was determined by qualitative radiological analysis. Fifteen patients who had undergone two CTs within one hospitalization were selected, and a DRR was generated from one CT. The radiologists were able to determine small differences in vertebral positioning between the DRR and the CT. In addition, radiologists determined that the application was clinically useful for visualizing certain radiographic entities that were not well represented by the CT. Further analysis of this program may involve standardization with a phantom to quantitatively compare DRR and CT images.
Speed comparison
This extension allows for the rapid generation of DRRs with a highly customizable graphical user interface. Example runs of the program generated a DRR in an average of 1.023 s. The extension is freely available and can be downloaded directly from 3D Slicer through its Extension Manager. The source code is available on GitHub.[11] Imaging data for testing purposes were acquired from The Cancer Imaging Archive.
DISCUSSION
As medical technology becomes more focused on imaging, the need to visualize anatomy in different ways becomes increasingly important. We propose DRR generator, which is a free extension for 3D Slicer, an open-source imaging software, that can produce a DRR from a CT image. A DRR can be manually compared to an X-ray, which provides utility to physicians wanting to compare changes that occurred between the pre-operative and operative imaging of the patient. The DRR can also be used on its own to view anatomy not visible on X-ray or to reduce the noise associated with X-rays.
DRR generator is novel in that it can generate DRRs while depending only on open-source software as well as providing a graphical user interface for adjusting the image. This extension generates a fast and accurate DRR based on CT image input. There are many applications for this extension, including vertebral position during surgery and catheter reconstruction during brachytherapy.
Further advancements in DRR generator may involve optimization of the speed at which DRRs are generated, as well as expansion of the functionality to work with other imaging modalities, such as MRI data.
CONCLUSION
A 3D Slicer extension was written that allows for rapid and highly customizable generation of DRRs. This extension should increase the accessibility of DRR generation to researchers and clinicians.
Distribution
DRR generator can be downloaded as an extension for 3D Slicer 4.11.0 and above. Source code is available on GitHub. Users are encouraged to contact the authors to share any improvements or bugs.
Licensing and collaboration
3D Slicer and DRR generator share a BSD-style open-source license that allows unrestricted use of the software for research, clinical, and commercial projects. Collaboration is encouraged and any further advancements or bug fixes may be integrated into the extension.
Declaration of patient consent
Patient’s consent not required as patients identity is not disclosed or compromised.
Financial support and sponsorship
Nil.
Conflicts of interest
There are no conflicts of interest.
References
- Fast Generation of Digitally Reconstructed Radiograph through an Efficient Preprocessing of Ray Attenuation Values San Diego, CA: Proceedings of SPIE; 2016.
- [Google Scholar]
- An image correlation procedure for digitally reconstructed radiographs and electronic portal images. Int J Radiat Oncol Biol Phys. 1995;33:1053-60.
- [CrossRef] [Google Scholar]
- Real-time DRR generation using cylindrical harmonics. In: Lecture Notes in Computer Science. 2002. p. :2489. Medical Image Computing and Computer-Assisted Intervention, 5th International Conference
- [Google Scholar]
- A Novel Fast DRR Generation Method by Reassembling Previously Generated Projection Blocks Las Vegas, NV: Institute of Electrical and Electronics Engineers; 2016. p. :272-5.
- [Google Scholar]
- GPU Accelerated generation of digitally reconstructed radiographs for 2-D/3-D image registration. IEEE Trans Biomed Eng. 2012;59:2594-603.
- [CrossRef] [PubMed] [Google Scholar]
- 3D slicer: A platform for subject-specific image analysis, visualization, and clinical support. In: Intraoperative Imaging and Image-Guided Therapy. New York: Springer; 2014. p. :277-89.
- [CrossRef] [Google Scholar]
- The Visualization Toolkit: An Object-Oriented Approach to 3D Graphics. New York: Kitware Inc; 2006.
- [CrossRef] [PubMed] [Google Scholar]
- An easy and novel method for safer brachytherapy: Real-time fluoroscopic verification of high-dose-rate 192Ir source position using a flat-panel detector. J Radiat Res. 2019;60:412-5.
- [CrossRef] [PubMed] [Google Scholar]
- Fast, automatic, and accurate catheter reconstruction in HDR brachytherapy using an electromagnetic 3D tracking system. Med Phys. 2015;42:1227-32.
- [CrossRef] [PubMed] [Google Scholar]
- CT imaging based digitally reconstructed radiographs and their application in brachytherapy. Phys Med Biol. 2000;45:2787-800.
- [CrossRef] [PubMed] [Google Scholar]
- Available from: https://www.github.com/lancelevine/SlicerDRRGenerator [Last accessed on 2020 Oct 10]